GBIF has reached 1 billion occurrences which is, of course, something to celebrate:
#GBIF1billion has arrived! Merci beaucoup, @Le_Museum @INPN_MNHN et @gbiffrance!
— GBIF (@GBIF) July 4, 2018
Thanks and congratulations, too, to the 1,217 data publishers and 92 participants who make the GBIF network go! More details to follow Thursday (champagne doesn't drink itself)… pic.twitter.com/xQ2f5fIt2x
An achievement on this scale represents a lot of work by many people over many years, years spent developing simple standards for sharing data, agreeing that sharing is a good thing in the first place, tools to enable sharing, and a place to aggregate all that shared data (GBIF).
So, I asked a question:
So I guess the real #GBIF1billion question is what can we do with a billion data points that we couldn't do with, say, a hundred million? Does more data simply mean more of same kind of analyses, or does it enable something new (and exciting)? @GBIF
— Roderic Page (@rdmpage) July 4, 2018
My point is not to do this:
Hey, don't spoil the party!
— Dimitri Brosens (@Dimibro) July 4, 2018
Rather it is to encourage a discussion about what happens when we have large amounts of biodiversity data. Is it the case that as we add data we simply enable more of the same kind of science, only better (e.g., more data for species distribution modelling), or do we reach a point where new things become possible?
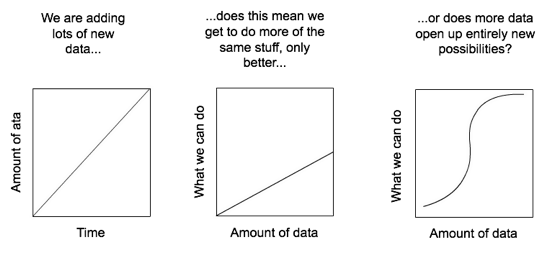
To give a concrete example, consider iNaturalist. This started out as a Masters project to collect photos of organisms on Flickr. As you add more images you get better coverage of biodiversity, but you still have essentially a bunch of pictures. But once you have LOTS of pictures, and those are labelled with species names, you reach the point where it is possible to do something much more exciting - automatic species identification. To illustrate, I recently took the photos below:
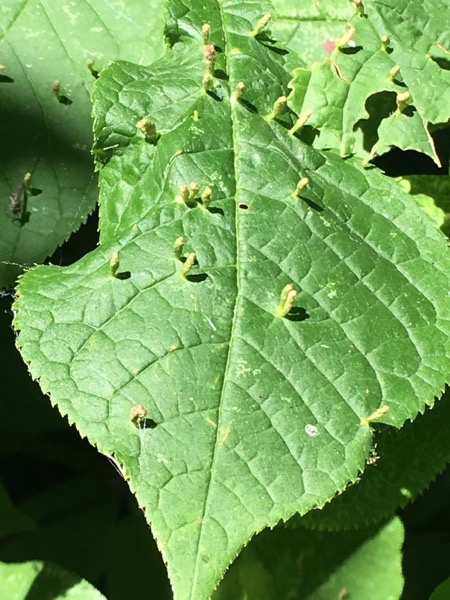

Note the reddish tubular growths on the leaves. I asked iNaturalist to identify these photos and within a few seconds it came back with Eriophyes tiliae, the Red Nail Gall Mite. This feels like magic. It doesn't rely on complicated analysis of the image (as many earlier efforts at automated identification have done) it simply "knows" that images that look like this are typically of the galls of this mite because it has seen many such images before. (Another example of the impact of big data is Google Translate, initially based on parsing lots of examples of the same text in multiple languages.)
Okay, but then not sure I see what you're looking for. Why would 1 billion, as opposed to, say, 100 million, mean a paradigm shift? Do you have any (even hypothetical) answers to suggest yourself?
— Leif Schulman (@Leif_Sch) July 5, 2018
The "1 billion" number is not, by itself, meaningful. It's rather that I hope that while we're popping the champagne and celebrating a welcome, if somewhat arbitrary milestone, I'm hoping that someone, somewhere is thinking about whether biodiversity data on this scale enables something new.
Do I have answers? Not really, but here's one fairly small-scale example. One of the big challenges facing GBIF is getting georeferenced data. We spend a lot of time using a variety of tools and databases to convert text descriptions one collection localities into latitude and longitude. Many of these descriptions include phrases such as "5 mi NW of" and so we've developed parsers to attempt to make sense of these. All of these phrases and the corresponding latitude and longitude coordinates have ended up in GBIF. Now, this raises the possibility that after a point, pretty much any locality phrase will be in GBIF, so a way to georeference a locality is simply to search GBIF for that locality and use the associated latitude and longitude. GBIF itself becomes the single best tool to georeference specimen data. To explore this idea I've built a simple tool on glitch https://lyrical-money.glitch.me that takes a locality description and geocodes it using GBIF.

You paste in a locality string and it attempt to find that on a map based on data in GBIF. This could be automated, so you could imagine being able to georeference whole collections as part of the process of uploading the data to GBIF. Yes, the devil is in the details, and we'd need ways to flag errors or doubtful records, but the scale of GBIF starts of open up possibilities like this.
So, my question is, "what's next?".