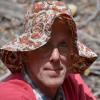
There's still time (to 31 March) to enter a dataset in the 2020 Darwin Core Million, and by way of encouragement I'll celebrate here the best and worst Darwin Core datasets I've seen.
The two best are real stand-outs because both are collections of IPT resources rather than one-off wonders.
The first is published by the Peabody Museum of Natural History at Yale University. Their IPT website has 10 occurrence datasets totalling ca 1.6M records updated daily, and I've only found minor data issues in the Peabody offerings. A recent sample audit of the 151,138 records with 70 populated Darwin Core fields in the botany dataset (as of 2020-03-18) showed refreshingly clean data:
- entries correctly assigned to DwC fields
- no missing-but-expected entry gaps
- consistent, widely accepted vocabularies and formatting in DwC fields
- no duplicate records
- no character encoding errors
- no gremlin characters
- no excess whitespace or fancy alternatives to simple ASCII characters
- 14 records with plant taxa mis-classified as animals
- 4 records with dateIdentified earlier than eventDate
- minor pseudo-duplication in several fields, e.g. "Anna Murray Vail; Elizabeth G. Britton" and "Anne Murray Vail; Elizabeth G. Britton" in recordedBy
- minor content errors in some entries, e.g. "tissue frozen; tissue frozen" and "|" (with no other characters in the entry).
The second IPT resource worth commending comes from GBIF Portugal and consists of 108 checklist, occurrence record and sampling event datasets. As with the Peabody resource, the datasets are consistently clean with only minor (and scattered) structural, format or content issues.
The problems appearing most often in these datasets are "double-encoding" errors with Portugese words and no-break spaces in place of plain spaces, and for both of these we can probably blame the use of Windows programs (like Excel) at the contributing institutions. An example of double-encoding: the Portugese "prôximo" is first encoded in UTF-8 as a 2-byte character, then read by a Windows program as two separate bytes, then converted back to UTF-8, resulting in the gibberish "prôximo". A large proportion of the no-break spaces in the Portugese datasets unfortunately occur in taxon name strings, which don't parse correctly and which GBIF won't taxon-match.
And the worst dataset? I've seen some pretty dreadful examples from around the world, but the UK's Natural History Museum sits at the top of my list of delinquent providers. The NHM offers several million records and a disappointingly high proportion of these have very serious data quality problems. These include invalid and inappropriate entries, disagreements between fields and missing-but-expected blanks.
Ironically, the NHM's data portal allows the visitor to select and examine/download records with any one of a number of GBIF issues, like "taxon_match_none". Further, for each record the data portal reports "GBIF quality indicators", as shown in this screenshot:
Clicking on that indicator box gives the portal visitor a list of the things that GBIF found wrong with the record (a list that overlaps incompletely with the list I can find with a data audit). I'm sure the NHM sees this facility differently, but to me it nicely demonstrates that NHM has prioritised Web development over data management. The message I get is
"We know there's a lot wrong with our data, but we're not going to fix anything. Instead, we're going to hand our mess as-is to any data users out there, with cleverly designed pointers to our many failures. Suck it up, people."In isolation NHM might be seen as doing what it can with the resources it has. In a broader context the publication of multitudes of defective records by NHM is scandalous. Institutions with smaller budgets and fewer staff do a lot better with their data — see above.